Correlation clustering is performed on databases and other large data sources to group together similar datasets, while also alerting the user to dissimilar datasets. This can be done perfectly in some graphs, while others will experience errors because it will be difficult to differentiate similar from dissimilar data. In the case of the latter, correlation clustering will help reduce error automatically. This is often used for data mining, or to search unwieldy data for similarities. Dissimilar data are commonly deleted, or placed into a separate cluster.
When a correlation clustering function is used, it searches for data based on the user’s instructions. The user will tell the program what to search for and, when it is found, where to place the data. This is normally applied to very large data sources when it would be impossible — or take too many hours — to search through the data manually. There can be either perfect clustering or imperfect clustering.
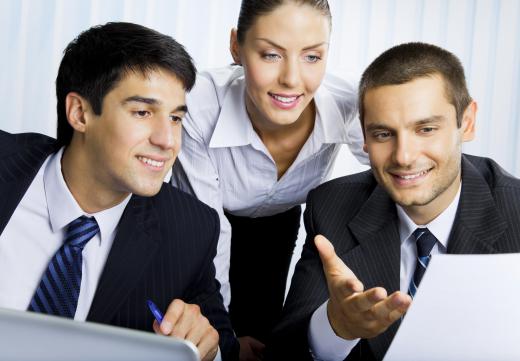
Perfect clustering is the ideal scenario. This means there are only two types of data, and one is what the user is looking for while the other is unneeded. All the positive, or needed, data are placed in one cluster, while the other data are deleted or moved. In this scenario, there is no confusion and everything works perfectly.
Most complex graphs do not allow perfect clustering, and are, instead, imperfect. For example, a graph has three variables: X, Y and Z. X,Y is similar, X,Z is similar, but Y,Z is dissimilar. The three variable clusters are so similar, however, that it is impossible to have perfect correlation clustering. The program will work to maximize the number of positive correlations, but this will still require some manual searching from the user.
In data mining, especially when dealing with large data sets, correlation clustering is used to group similar data with similar data. For example, if a business mined data for a large website or database and only wants to know about a specific aspect, it would take forever to search through all the data for that aspect. By using a clustering formula, the data will be set aside for proper analysis.
Dissimilar information is dealt with based solely on user instructions. The user can elect to send dissimilar data to different clusters, because the information may be useful for other projects. If the data are unneeded and are just wasting memory, then the dissimilar information is thrown out. In imperfect clustering, it is possible that some dissimilar information will not be thrown out, because it is so similar to the data for which the user is looking.